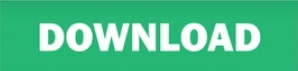
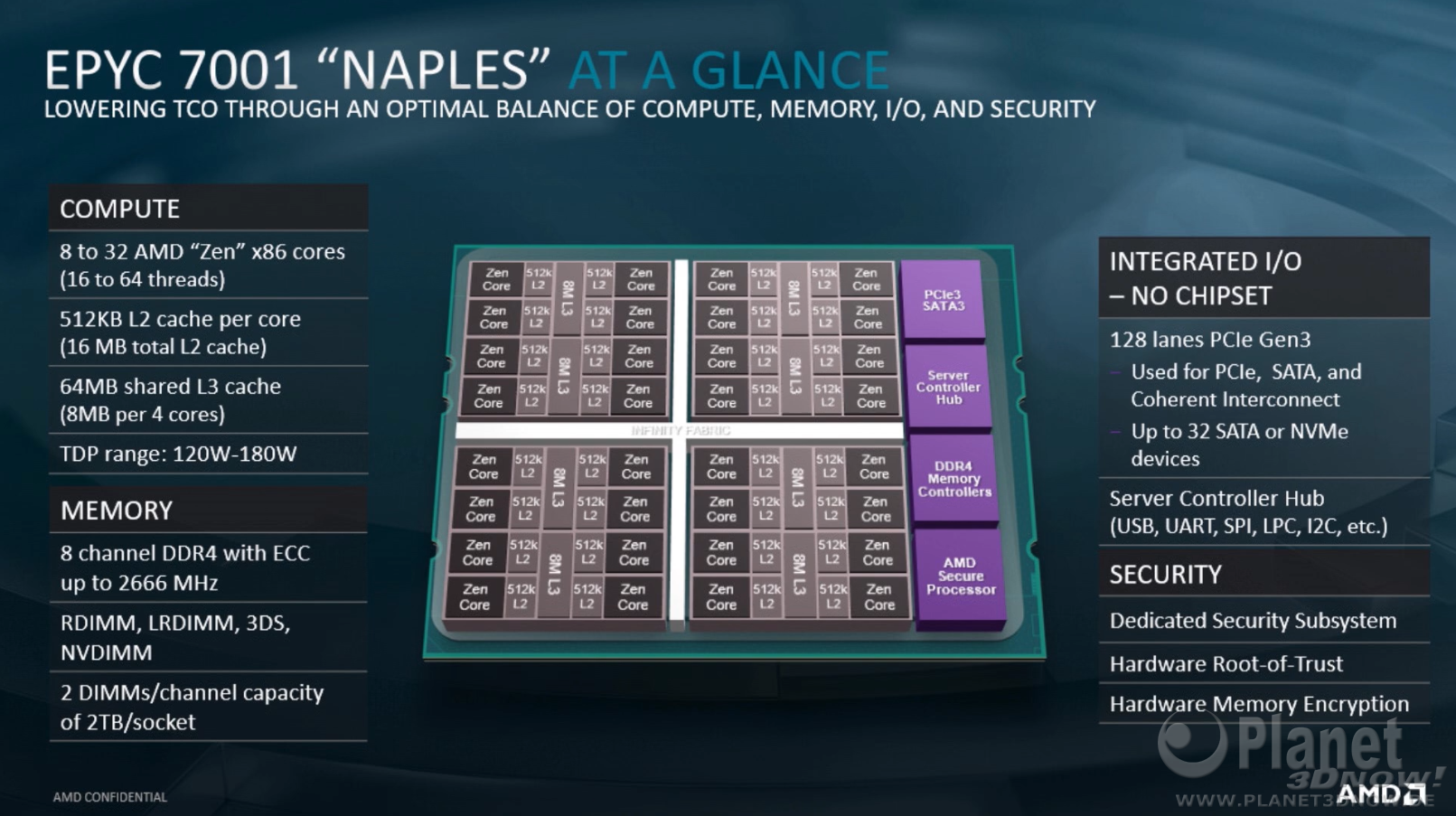
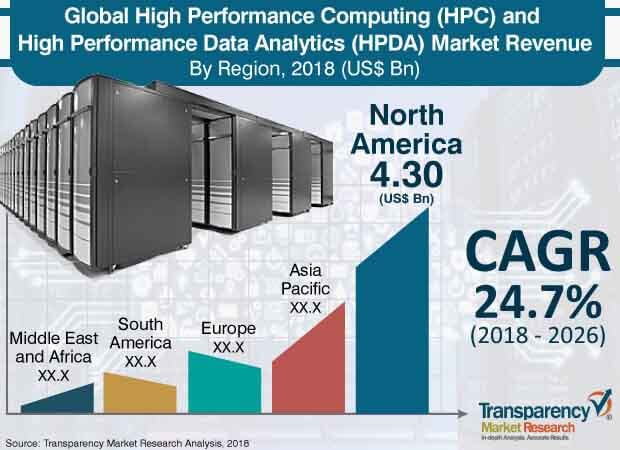
Hpc ai benchmark pdf#
We will select five speakers from session leaders and leading experts in academia, government, and industry for presentation.Īudience participation is a key success metric of this BOF, and 50% the time has been allocated for discussion.Download a PDF of the paper titled AIPerf: Automated machine learning as an AI-HPC benchmark, by Zhixiang Ren and 8 other authors Download PDF Abstract: The plethora of complex artificial intelligence (AI) algorithms and available (6) What is the impact of data and workload distributions among HPC, datacenter, edge and IoT on the system performance behaviors and workload characterization.Īll session leaders and invited speakers have strong experience in AI. (5) What are the metrics of evaluating different AI systems?
Hpc ai benchmark how to#
(4) How to build end-to-end application benchmarks without loss of the flexibility of micro and component benchmarks. (3) How to contribute the data sets as most of the owners consider data as confidential? (2) What are the essentials of real-world AI workloads and applications? How to specify the common requirements of the AI workloads only algorithmically in a paper-and-pencil approach? (1) What is the scalable benchmarking methodology other than creating a new benchmark or proxy for every possible AI workload? Together with participants from different communities, we will discuss the following issues. Now is the perfect time to start this project.
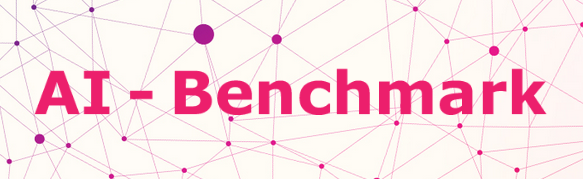
The aim of this BoF is to discuss how to build a comprehensive AI benchmark suite for HPC, data center, Edge, and IoT with an emphasis on data and workload distributions among them.
Hpc ai benchmark software#
Third, for co-design of software and hardware, we need simple but elegant abstractions that help achieve both efficiency and general-purpose. Second, for the sake of fairness, the benchmarks must include diversity of data and workloads. First, for the sake of conciseness, benchmarking scalability, portability cost, reproducibility, and better interpretation of performance data, we need understand what are the most time-consuming classes of unit of computation among big data and AI workloads.

However, complexity, diversity, frequently changed workloads, and rapid evolution of AI systems raise great challenges in benchmarking. Long Description: As architecture, system, data management, and machine learning communities pay great attention to innovative AI or machine learning algorithms, architecture, and systems, the pressure of benchmarking rises. The aim of this BoF is to discuss how to build a comprehensive AI benchmark suite across different communities with an emphasis on data and workload distributions among HPC, data center, Edge, and IoT. However, complexity, diversity, frequently changed workloads, and rapid evolution of AI workloads and systems raise great challenges in AI benchmarking. AIBench: Toward a Comprehensive AI Benchmark Suite for HPC, Datacenter, Edge, and IoTĪuthors: Jianfeng Zhan (Institute of Computing Technology, Chinese Academy of Sciences), Xiaoyi Lu (Ohio State University), Wanling Gao (Institute of Computing Technology, Chinese Academy of Sciences)Ībstract: As diverse communities pay great attention to innovative AI or machine learning algorithms, architecture, and systems, the pressure of benchmarking rises.
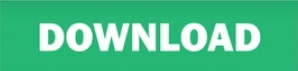